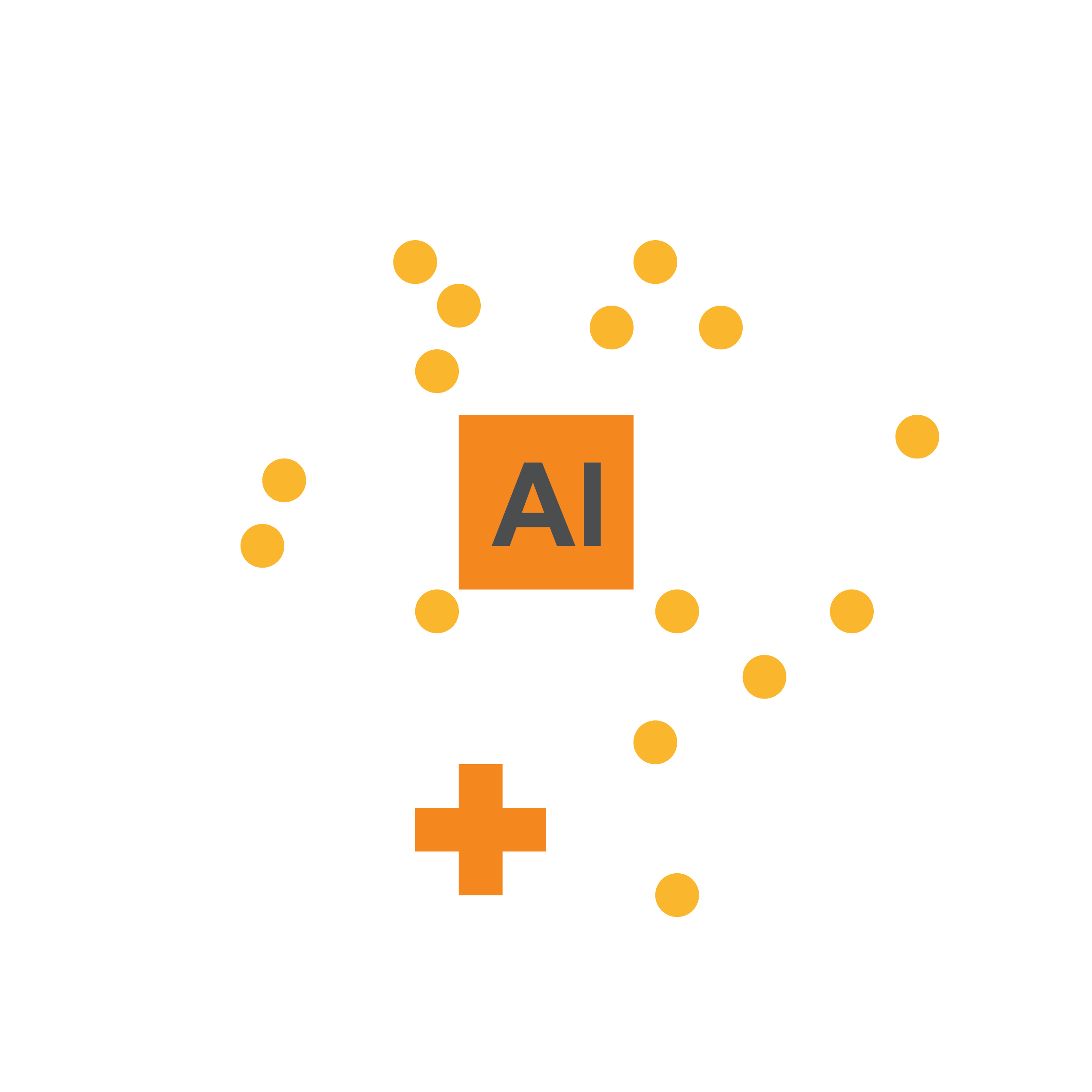
Automatic Detection of Myocardial Infarction with Artificial Intelligence
SLC-GAN: An Automated Myocardial Infarction Detection Model
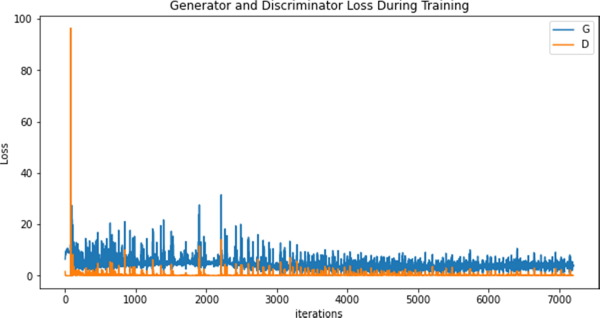
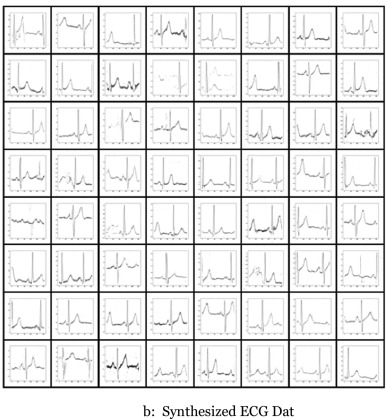
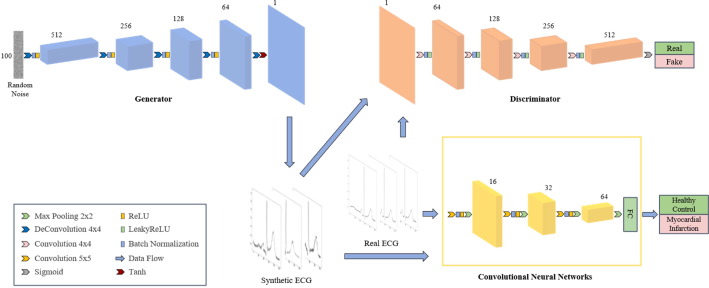
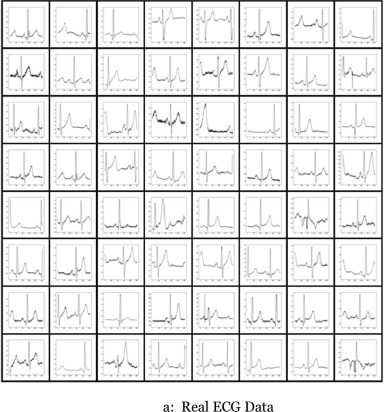
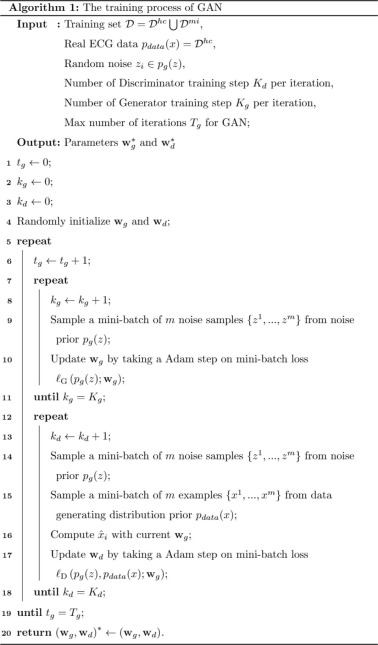
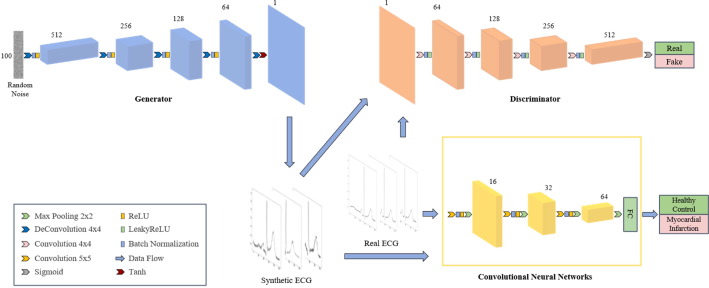
Automated myocardial infarction detection model
This paper proposes an automated myocardial infarction detection model — SLC-GAN, that synthesizes single-lead ECG data with high morphological similarity through Generative Adversarial Networks (GAN). It automatically detects MI using Convolutional Neural Networks (CNN) with real ECG data and synthetic ECG from the GAN.
The electrocardiogram (ECG) is a sophisticated tool for the diagnosis of myocardial infarction (MI). Deep learning approaches can support MI diagnosis based on ECG data. However, due to the privacy issue and the sensitive nature of ECG data, it is still challenging to use deep learning methods to access large amounts of ECG data for training. Insufficient data will lead to a decrease in diagnosis performance. Besides, most deep learning approaches are trained with multiple-lead ECG data, limiting the extension to portable single-lead ECG monitoring devices. Investigating automated single-lead ECG interpretation is a continuing concern in developing mobile and wearable ECG monitoring devices. This paper proposes an automated myocardial infarction detection model — SLC-GAN, that synthesizes single-lead ECG data with high morphological similarity through Generative Adversarial Networks (GAN). It automatically detects MI using Convolutional Neural Networks (CNN) with real ECG data and synthetic ECG from the GAN. The empirical results indicate that our new SLC-GAN method achieves impressive performance compared with other MI classification methodologies based on single-lead ECG from the PTB Diagnostic ECG Database. The MI classification accuracy of SLC-GAN reaches 99.06% with 5-fold cross-validation.
Publication
Li W, Tang Y M, Yu K M, et al. SLC-GAN: An Automated Myocardial Infarction Detection Model Based on Generative Adversarial Networks and Convolutional Neural Networks with Single-Lead Electrocardiogram Synthesis[J]. Information Sciences, 2022.

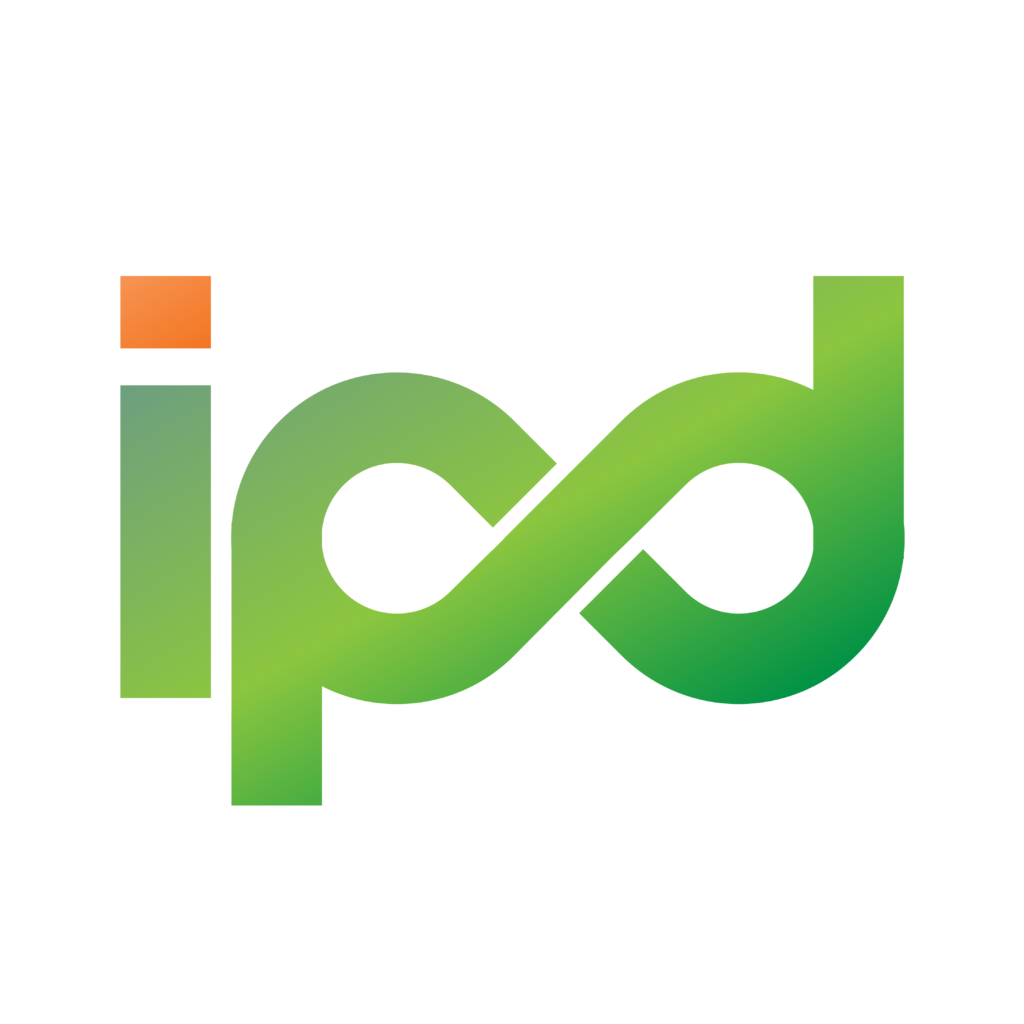
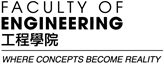
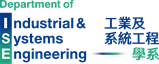
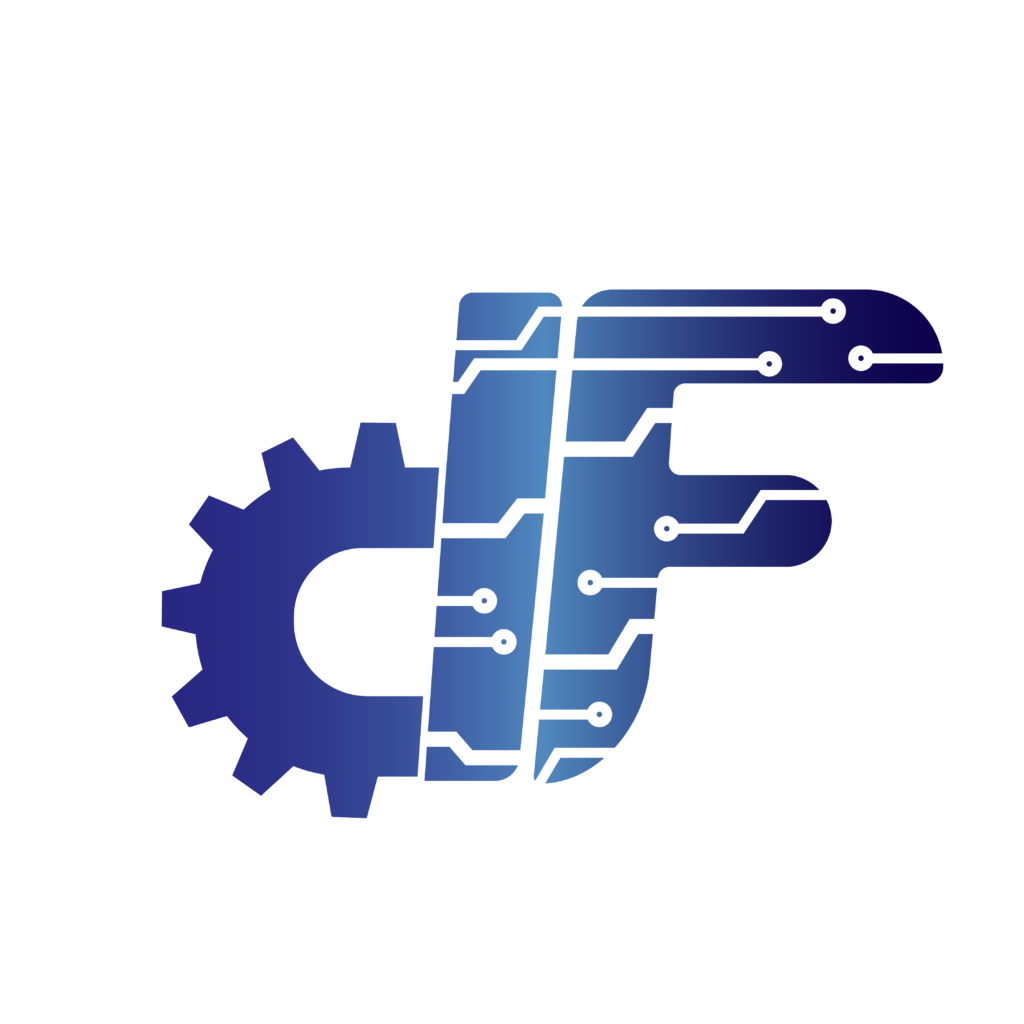